
6 Myths and misconceptions about data projects
As I considered how to promote my new book on driving data projects, I wanted to include myths and misconceptions that reinforce their value. I have experienced many of these in teams I’ve worked on or with. Data projects are not a static set of routines. It's a constantly evolving, open-to-innovation process.
Only 54 percent of organizations fully understand the value of project management, according to PMI's Pulse of the Profession™ report. That might explain, in part, why project success rates are so low: Less than two-thirds meet their original business intents.
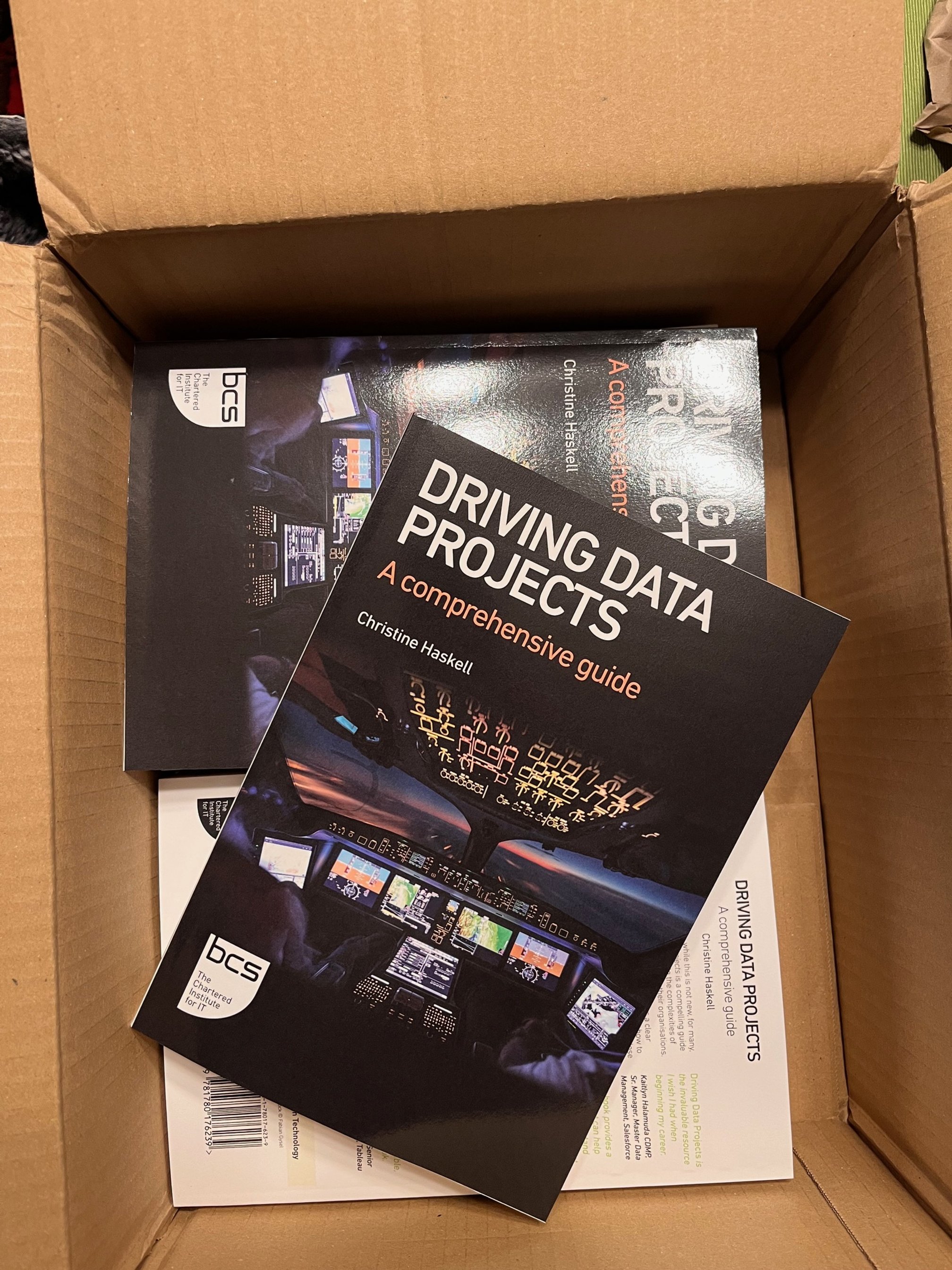
you get an author’s box!
I read somewhere that over 80% of adults want to write a book, but only 3% ever get to 'The End' of a draft. That means that 97% of people who want to write a book never finish.
Stats like that make accomplishments like getting my “author’s box” of books all the more rewarding.
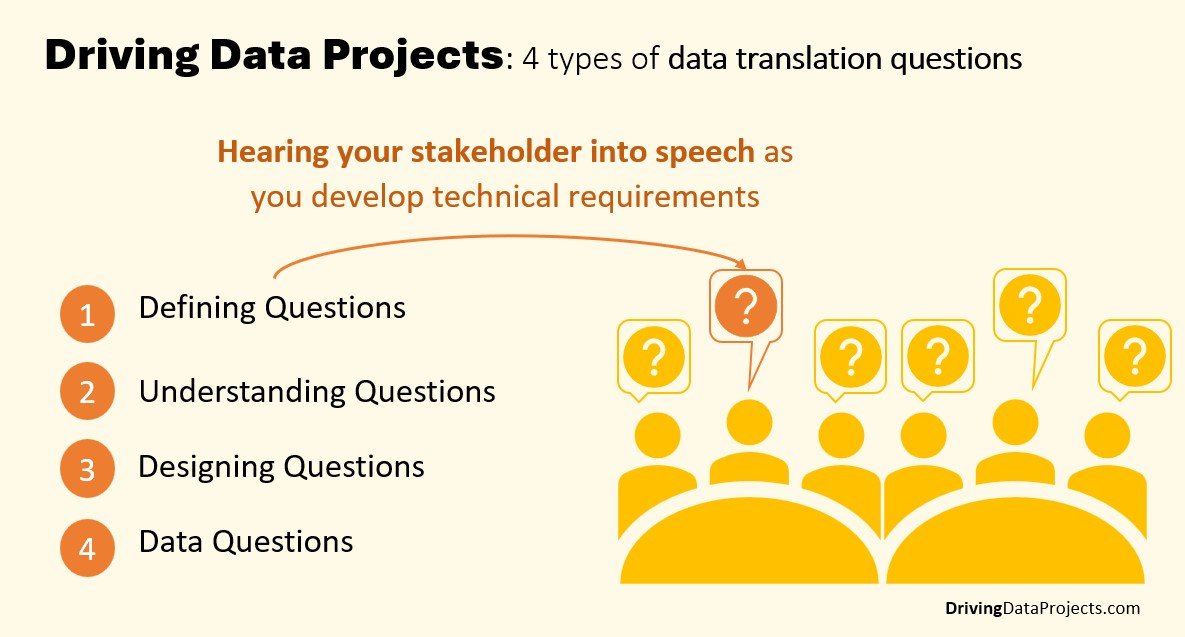
4 perspectives to drive effective data translation
When driving data projects, you will encounter business stakeholder challenges that often go unspoken. This is not always because people hold back but because they don't fully know how to vocalize their constraints.
If they can't directly address their requirement, chances are we can't either. To hear others' speech, we start by asking questions from different perspectives.
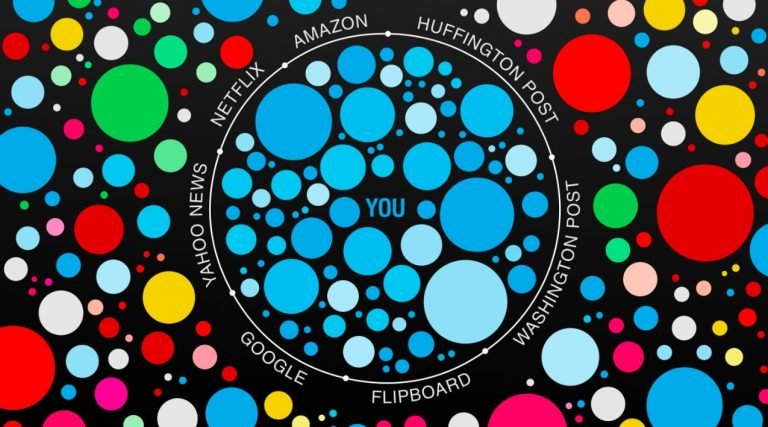
Data trend: from spreadsheets to algorithms
The transition from traditional spreadsheets to sophisticated algorithms in data management and analysis represents a significant evolution that has revolutionized how businesses process and leverage information. Algorithms have reshaped the landscape of data-driven decision-making.
When discussing algorithms and AI, we must discuss building AI Fluency in everyday people. EVERYONE needs to understand the impact and ethical considerations of sharing their data. While it’s likely too late with respect to data sharing, it’s important to understand the ethical and functional impacts of how AI is being applied to that data so that people can advocate for themselves.
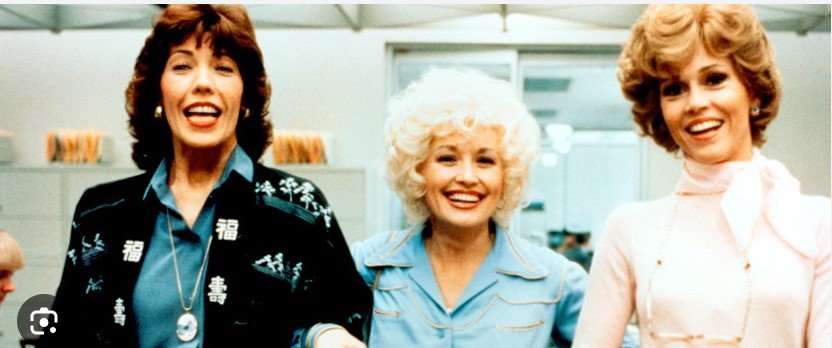
Rethinking Remote work: again
Virtual work has been going through a shift for over forty years. I know, it’s hard to believe that anything before the 2000s counts. But there were hopes and dreams for flexible hours and work-from-home initiatives as early as the 1970s—they were fringe, but they were there.
Considering what is happening in the world today, it is interesting to note that data is a great enabler of diversity, equity, and inclusion and a general concept of fairness and democratization. Data busts silos and flattens barriers between functions. As this distribution is occurring, there will also be some disruptions.
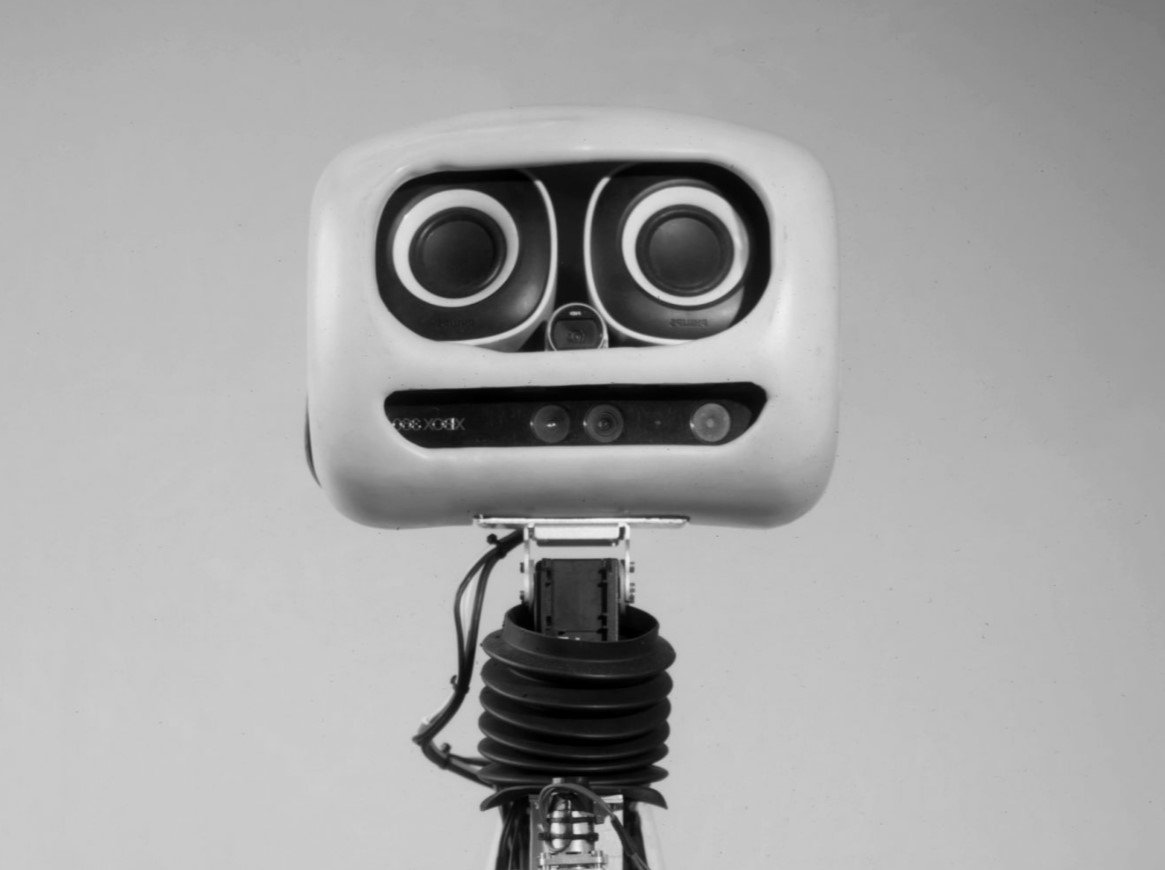
Who is pacing this race?
The idea of having a more freeform machine co-worker with whom we can ask natural language questions and who can anticipate our needs is just starting. However, this requires a higher level of digital fluency for us to really find a groove with these machine-coworkers and not be overtaken by them. As I came up in my career, we were taught to “automate ourselves out of a job” to do the next thing. Our skills, desire for change, interest in the business, and work dictated the pace. Today, the pace of automation may not be dictated by us. There is a real risk of jobs going away before we have thought through the next set of problems to solve. If we cannot see around corners, we have not considered our upcoming transition.
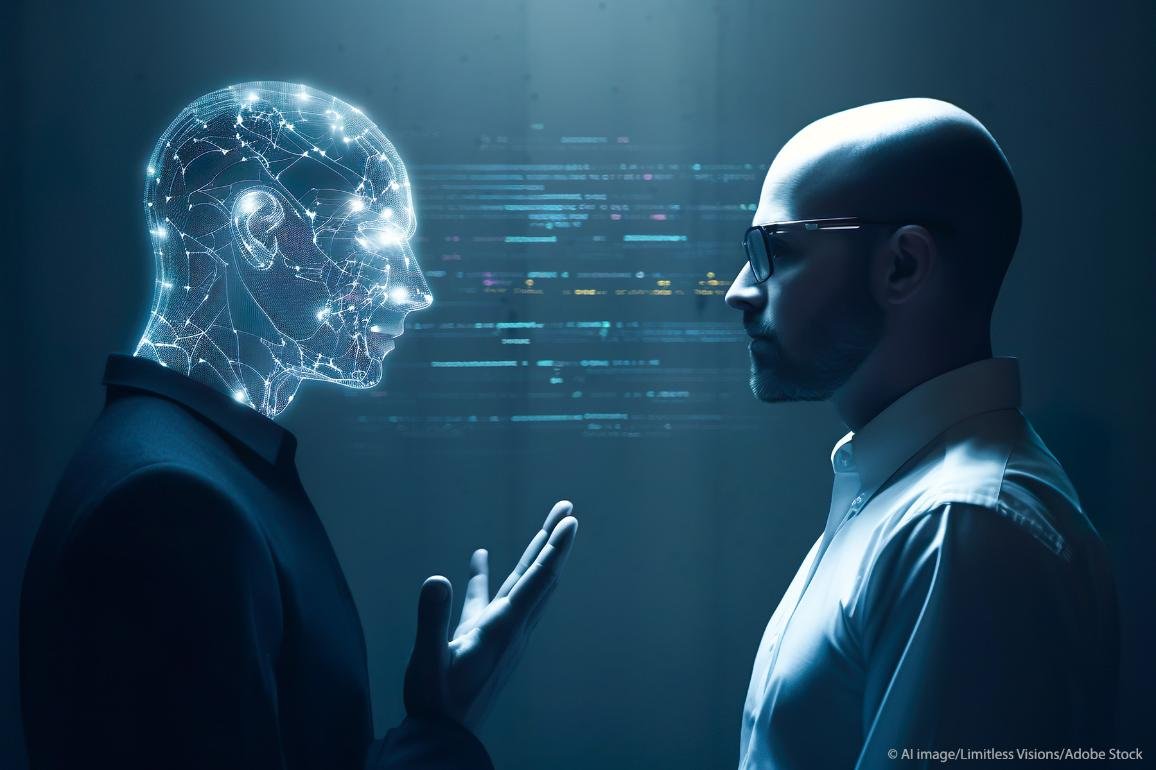
machine, my coworker
We often consider #digitaltechnologies like #dataplatforms #AI and #copilot features as tools. But if we're rethinking the #futureofwork and the future of careers and companies, it's helpful to think of these things as augmenting our efforts. For a copilot, in particular, it becomes a junior coworker or maybe a more senior coworker as the #AIskills get better.
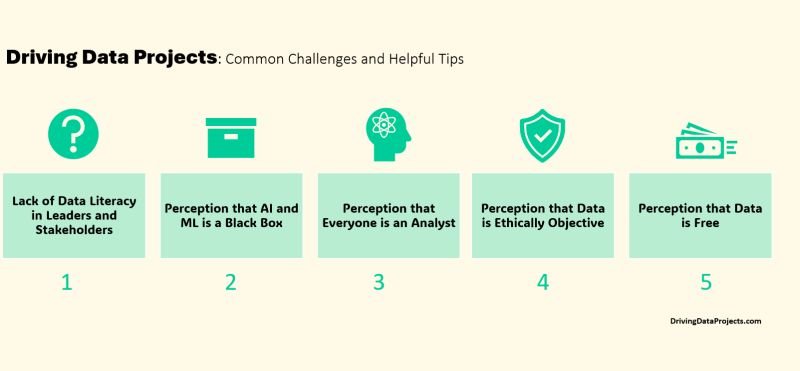
Tips and Challenges
As we continue to drive data projects, familiar challenges begin to present themselves. By observing, we can become better diagnosticians of systemic issues. Learn what to avoid and how to navigate them better.

Countdown: Book Excerpt Chapter 4
People who work in data management are particularly dedicated problem solvers. They are committed to the mission in a way that makes them want to make the initiative successful. Most examples featured in the book reflect what happens in a specific type of data project -- a team-based project with stakeholders recruited from across the organization, including outside partnerships.
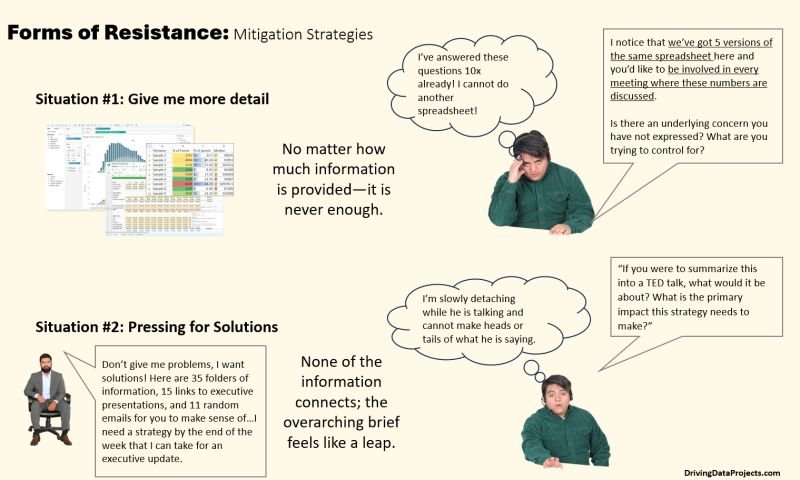
Resistance and Mitigation Strategies
Change management wouldn’t be so hard if it weren’t for…the people. Open issues or objections left unresolved today cost time down the road. Suppose work starts before these concerns are mitigated. Stakeholders might get frustrated or begin to hold back their participation. Work produced might have difficulty getting implemented. Buy-in realizes impact.
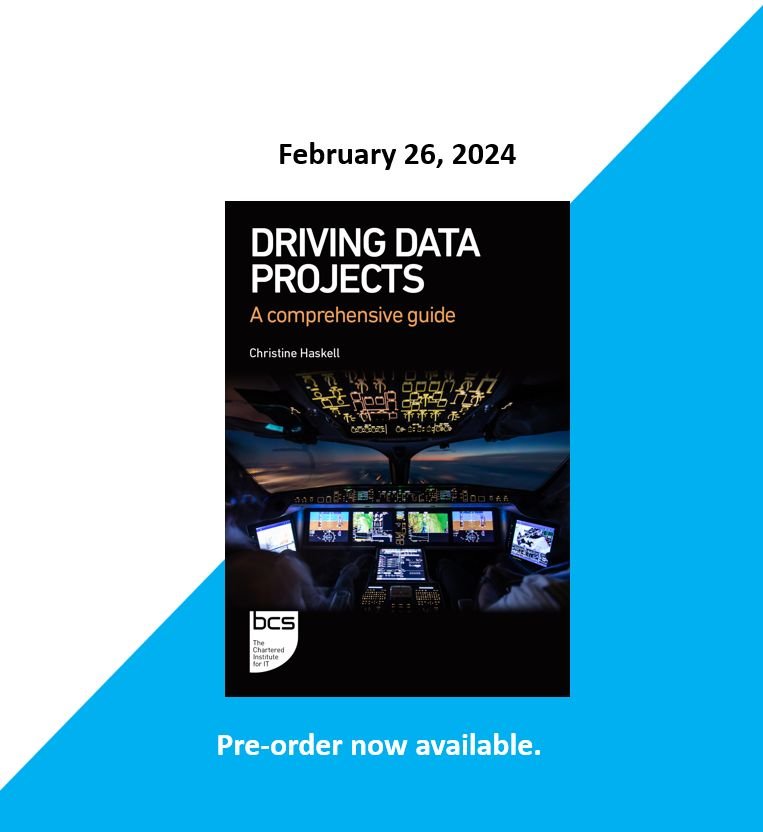
Countdown: Book Excerpt Chapter 3
Until an organization is willing to invest in its data capabilities, aligning data resources to answer complex business questions will be like riding a bicycle to chase a Formula One racer and never catching up. Scoping project opportunities well is about building enough trust to eventually scale resources. While a single project manager can accomplish some initiatives, most data projects require multi-disciplinary resources to execute.
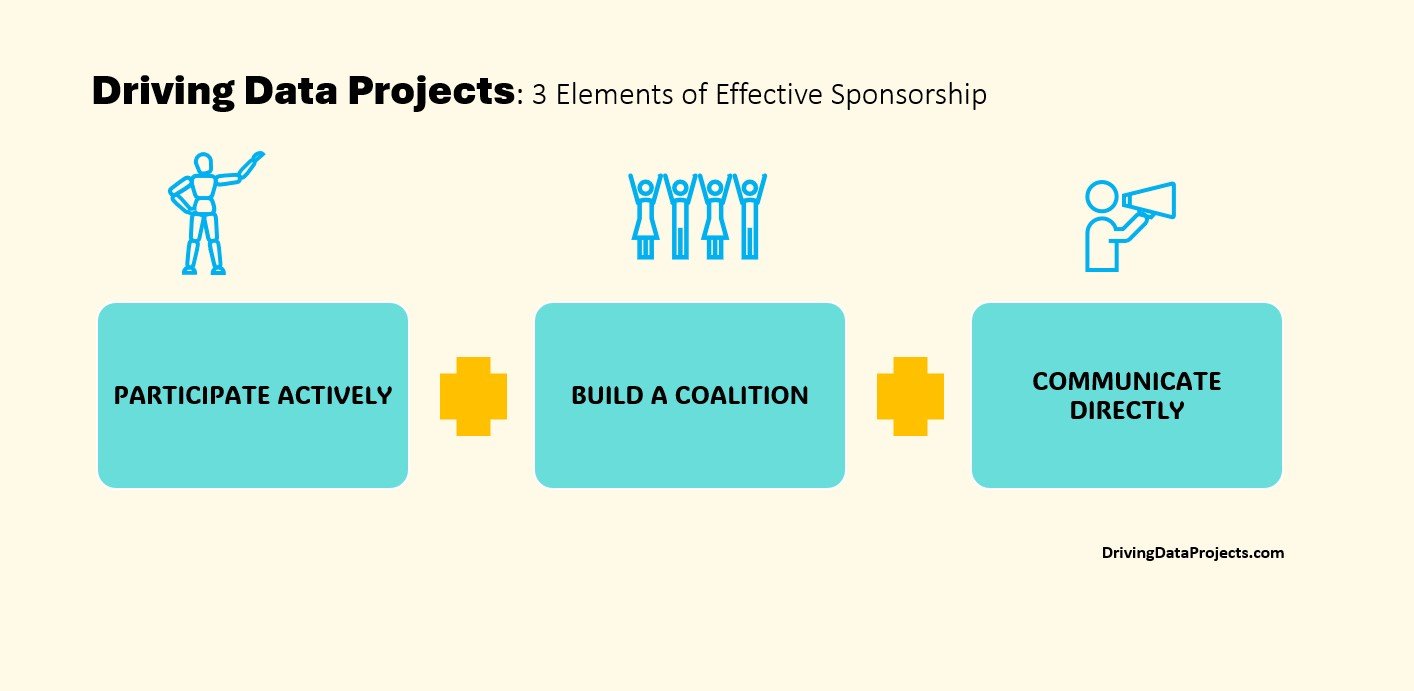
3 Elements of Effective Sponsorship
A popular misconception of senior leadership is that effective executive sponsorship is a clearly understood skill. Many assume executives receive developmental feedback about becoming effective sponsors. Sadly, there is little training on sponsorship from middle management on up.